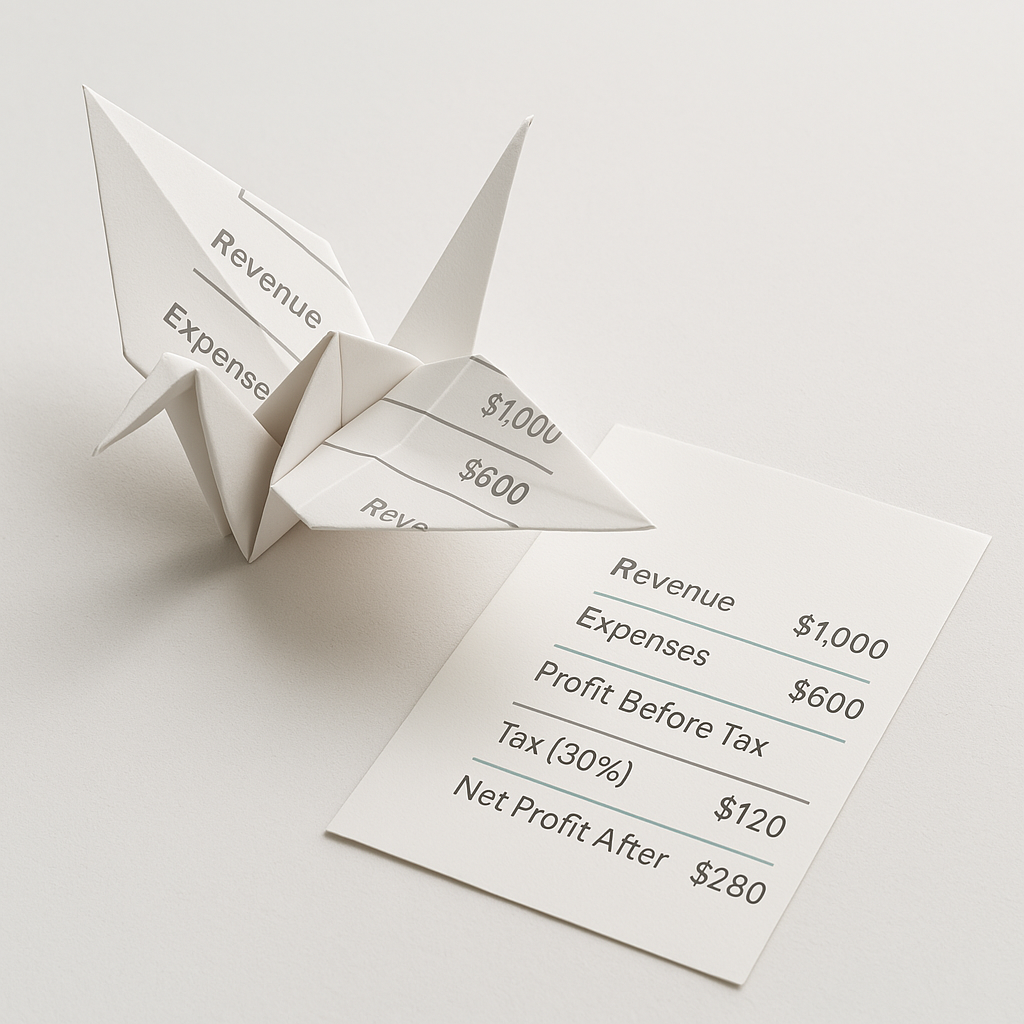
How Finance Can Keep Up in the Age of AI

Finance Is the Source of Truth — But Can't Move Fast Enough to Deliver It
You know how sales teams are now using AI to qualify leads instantly, prioritise deals with the highest close probability, and forecast revenue in real time?
Or how marketing can A/B test campaigns live, track conversions across channels by the hour, and get generative insights about what's driving engagement — while the campaign's still running?
Or how operations teams are using predictive analytics to anticipate supply chain risks days before they hit, automatically rerouting logistics to avoid delays?
Meanwhile, finance is waiting for the books to close — and the rest of the business is already making the next set of decisions.
By the time the numbers land, the moment they describe has already passed. Want to know your actuals? Hold tight. Need a full business forecast? Let us wrangle Excel first. And don’t even ask for a marketing ROI analysis — not until next quarter.
It’s not a talent problem. It’s not a motivation problem. It’s a design problem.
Finance was built for a different era — one defined by batch thinking and calendar cadence. A world where delays were acceptable, as long as the numbers were right.
But AI is changing that tempo.
Agents are learning to operate at machine speed. They're resolving customer cases, qualifying leads, and optimising marketing campaigns — without waiting for a prompt. Triggered by data changes, business rules, or API signals, they act autonomously across systems. The business is speeding up — exponentially.
And finance?
It’s still stuck in first gear.
If finance can’t change gears, it could cost finance its seat at the strategy table. And businesses without integrated finance will be outpaced by those who’ve already made the leap.
Get the Latest Insights from Accurate Digits
How We Got Here
Finance hasn’t stood still.
We’ve gone from paper ledgers to ERPs, from siloed systems to global platforms, from Excel models to integrated forecasting tools in the cloud. Automation has streamlined routine tasks. Dashboards have brought visibility to key metrics. And closes now happen faster than ever before.
But for all that progress, one thing hasn’t changed: the rhythm.
The business still moves on a monthly beat — and in many ways, that cadence works. Humans need time to absorb information, spot patterns, and implement change. A monthly cycle gives space to reflect, align, and act. It works for the business. And, truthfully, it works for finance.
Because finance was designed for precision, not haste.
We don’t move fast because we can’t. We move carefully because we must.
The numbers we produce carry consequences — for leadership, for markets, for regulation. Mistakes don’t just cost time. They cost trust. And so, our systems are built to prioritise certainty and accuracy.
This cautious mindset has served us well.
But it also means finance is slow to adopt what feels unproven.
We’re not the early adopters — and with good reason.
Sales and marketing? Their risk appetite is high — not because the stakes are low, but because the downside is manageable. They’ll trial new CRM tools, pilot AI powered sales enablement apps, and experiment with AI-generated content — all in pursuit of generating more leads, faster sales cycles, and better conversion. If something doesn’t work, they move on.
Which is why the difference in Generative AI adoption isn’t surprising.
According to McKinsey’s 2025 “The State of AI report”, 42% of sales and marketing functions have integrated Generative AI. In finance? Just 11%. That’s a fourfold gap. Not because finance lacks capability, but because it’s governed by a different risk-reward equation.
But that deliberate pace — the one that’s served finance so well — is about to meet its biggest test yet. Because if Generative AI feels transformative, you haven’t seen anything yet. There is a new way of working — one that moves exponentially faster than anything we’ve seen before.
What’s Next: From Prompts to Purpose
Generative AI has been transformative. It drafts analysis, suggests insights, writes content, and supports ideation across every function. And it's getting better fast — improving in reasoning, accuracy, and its ability to process multimodal inputs like text, images, audio, and video. The surface area of what AI can touch is expanding by the day.
But for all that progress, Generative AI is still fundamentally reactive. It waits for prompts. It relies on human guidance. It’s powerful — but narrow. Great at discrete tasks like drafting a memo, analysing a dataset, or summarising a meeting.
What it can’t do is manage complexity. It doesn’t initiate, orchestrate, or adapt in real time. That’s where the next evolution begins.
Enter Agentic AI.
What is Agentic AI?
If generative AI was the copilot, Agentic AI is the digital labour-force.
It’s not necessarily smarter — it’s just structured differently. Systems built not to assist humans task by task, but to act with purpose, solve with autonomy, and operate at the speed of machine.
It’s powered by the convergence of stronger foundation generative AI models — now more accurate, better at reasoning, and capable of processing multimodal inputs like text, images, audio, video, and live search — and by the orchestration layer that connects them. These aren’t just tools that wait for instructions. They’re agents — digital workers designed to take initiative. They can start tasks on their own, gather information, make decisions, and complete multi-step processes. Humans still stay involved at key points — to review, approve, or adjust — but much of the heavy lifting can now happen automatically, behind the scenes.
This isn’t just about “using AI” anymore. It’s about rethinking how work gets done — with AI not just helping along the way, but driving the process itself.
That’s the shift: from asking AI to do something, to AI knowing what to do — and doing it.
That’s Agentic AI
What Agentic AI Can Already Do
Take Salesforce’s Agentforce. A customer submits a billing dispute — but instead of routing it to a service rep, the AI agent gets to work. It pulls the customer’s transaction history, checks against policy, validates the claim, and resolves the issue — all autonomously, without waiting for a prompt. It’s not just answering questions. It’s closing tickets.
Or consider research. With tools like ChatGPT’s Deep Research, an agent can scan hundreds of sources, extract relevant insight, weigh conflicting viewpoints, and build a coherent, structured report — the kind you'd normally ask a junior analyst to prepare. And it adapts as it learns: changing its approach based on what it finds.
They’re not just helpful — they’re remarkably capable at completing complex tasks, start to finish, without being handheld.
The Evolving Landscape of Enterprise AI Agents
So far, most AI agents have been specialists — focused on doing one task really well. They’re fast, accurate, and efficient at things like drafting emails, checking documents, or updating systems. But what’s coming next is bigger.
We’re about to see the rise of orchestrator agents — AI agents that don’t just complete tasks, but manage other agents. Think of them as digital project managers: coordinating multiple specialised agents, assigning work, tracking progress, and driving outcomes across complex, multi-step workflows. And beyond that? A world where agents from different companies interact directly — negotiating terms, managing transactions, even making decisions autonomously. The pace of this evolution is accelerating. And the organisations that understand where it’s heading will be best placed to lead it.
The Problem – Integrating AI Agents into Companies
AI has reached a level of speed and capability that’s game-changing — but unlocking its value inside real-world organisations remains hard. Why? Because AI and human teams operate on fundamentally different terms.
Two core challenges define this tension:
- AI is incredibly fast.
What takes a human hours or days, an AI model can often complete in minutes — if it has the right context. Its throughput, memory, and retrieval speed far exceed human capacity. - AI lacks context.
Humans effortlessly understand unspoken cues — hierarchy, nuance, physical environments. AI models struggle with this. Without clear, structured context, they underperform or fail silently.
Organisations that want to benefit from AI must bridge this divide: harnessing AI’s speed while embedding the rich context it needs to operate effectively.
So why are sales, marketing, and operations racing ahead?
Because Generative AI plugged straight into systems that were already wired for speed — real-time data flows, API-connected apps, and feedback loops everywhere. Much of their “human context” was already codified: every customer interaction, every click, every conversion tracked in the CRM. The agents didn’t need to guess — they just got to work.
Finance has cloud tools too — modern ERPs, forecasting platforms, even copilots emerging within them. Helpful? Sure. Transformative? Not yet. These copilots operate in isolation, locked inside structured systems and far from the messy, manual workflows that still define how lots of finance actually gets done.
Nowhere is that clearer than month-end close. It’s a spaghetti of spreadsheets, manual reviews, and gut-feel decisions. Context lives in people’s heads and in last-minute meetings — not in the system. AI agents can’t accelerate what they can’t access. And right now, too much of finance still runs outside the loop.
The Risk
Sales, marketing and operations are already racing ahead, experimenting with AI agents that, for now, are mostly single-task specialist. But that won’t last.
Soon, we’ll begin to see networks of agents working together —coordinating across workflows, making decisions on the fly and allocating resources dynamically. These agents won't just execute tasks. They’ll be asked to prioritise, weigh trade-offs, and drive outcomes.
And here’s the catch: those decisions will only be as good as the financial context they’re built on.
But without financial context, those trade-offs risk being made in a vacuum. And here lies the issue: today's finance processes are built around monthly cycles. When key decisions rely on data that’s weeks out of date, insights arrive too late to matter. AI agents end up optimising a silo, fast but disconnected from the bigger picture.
Finance—the function uniquely positioned to provide comprehensive visibility into how resources flow, risks accumulate, and value is created—risks being sidelined simply because its workflows aren't in sync with the speed at which the business operates.
If finance doesn't accelerate its own tempo, the CFO’s strategic influence will diminish, weakening finance’s voice at the leadership table.
More critically, businesses that fail to modernise finance will fall behind those that do. With real-time infrastructure, AI agents can learn not twelve times a year, but twelve times an hour. They iterate faster, optimise better, and innovate continuously — compounding into an advantage that quickly becomes impossible to catch.
The Opportunity
In an agentic world, the size of a company will have less influence on market dominance. Why would it, when digital labour can scale instantly from zero to infinity, traditional advantages of scale diminish rapidly. Instead, success will favour organisations that learn, adapt, and act swiftly.
The true competitive advantage won't come from size, it will come from context: how well a company understands its environment, and how quickly it can turn that understanding into action.
This presents finance with a transformative opportunity. Finance must evolve to facilitate iterative decision making. Finance must become the engine that powers organisational agility and flow.
Enter the CFO: the Chief Flow Officer, a fundamentally reimagined mandate for finance leadership.
This new role shifts the finance function from reactive reporting to proactive enablement by:
Accelerating enterprise-wide decision-making across the enterprise through real-time insight and forecasts.
Surfacing trusted data, not at month-end, but in the moment.
Embedding economic context into every decision point, AI agent, and workflow across the organisation at exactly the right time to ensure strategic alignment and optimised resource allocation.
Finance becomes more than a checkpoint. It becomes the north star – the grounding force that guides a fast-moving organisation.
The playbook
The shift to an AI-powered world isn’t theoretical — it’s already underway. And finance can’t afford to sit on the sidelines. To stay relevant, finance teams need to move faster, deliver insight continuously, and embed economic context into decisions across the business.
That’s where this playbook comes in. It’s not about some distant future — it’s a two-speed plan: what to do to Thrive Now, and how to get Future Ready. One part helps you perform today. The other prepares you for what’s coming next. Because in a world moving at machine speed, the only way to keep up is to change how we work — structurally, systematically, and strategically.
Thrive now
To meet today’s pace, finance needs to move faster and respond sooner. You don’t need to build from scratch —the tools are already available. With the right steps, you can start improving responsiveness now. Here’s where to begin:
Lay the foundations
Modern finance runs on modern infrastructure. Focus on cloud, data, and systems to build a faster, more responsive core.
- Know your tech roadmap
Get clear on your core platforms — ERP, CRM, planning tools, and more. Prioritise modern, cloud-first systems that can evolve with you. Don’t invest in fixing processes tied to platforms heading for retirement. - Clean your data
Poor data quality ruins even the best systems. Use today’s AI tools to categorise, standardise, and spot inconsistencies — and back it with strong processes that keep your data accurate and reliable. - Keep improving
Cloud platforms improve constantly — so should your workflows. Identify processes not utilising the platform's latest features and move them forward, step by step.
Start Applying AI
In parallel, start putting AI to work as a practical way to boost output and reduce friction today.
- Boost productivity with generative AI
Use tools like ChatGPT or Microsoft Copilot to supercharge employee productivity, speed up analysis, and reduce repetitive work. - Tap specialist AI solutions
Find AI-powered tools that integrate with your accounting applications and workflows to help automate manual processes directly.
These steps are just to live in the now, to keep up with other functions moving faster and to improve finance’s responsiveness to the increasing demands of the business.
📢 Looking for practical tools to speed up your financial reporting workflows?
At Accurate Digits, we're helping finance teams apply AI to real-world reporting challenges — cutting review time, improving accuracy, and unlocking faster decision-making.
Learn more here: www.accuratedigits.com
Future ready
Future Ready means seeing beyond today. It's anticipating where the puck will be and meeting it there. Today's AI moves fast, but real value only emerges when AI has the rich, human-generated context it needs. You need to start building that context today, so your business can deploy AI agents seamlessly tomorrow.
The steps outlined in the Thrive Now phase lay the groundwork for becoming future ready. By moving from a tangle of spreadsheets to purpose-built, cloud-native solutions, you begin capturing rich data in a structured, systematic way. This not only improves current workflows — it also positions you to benefit early as cloud providers and their partners develop AI agents that can build on that foundation.
Here are additional actions you should start to consider to be Future Ready:
Reduce human friction
Human interaction slows processes and kills AI advantage of machine speed. Minimise it by:
- Documenting tribal knowledge in wikis, policies, and procedures—AI thrives on documented context.
- Replacing manual reviews and approvals with criteria-based pre-approvals.
- Replacing meetings that generate tribal knowledge with workflows capturing insights automatically.
- Leveraging AI note-taking tools not just for efficiency, but as future AI context.
Design processes for AI
Structure your workflows for AI’s strengths—turn judgement calls into repeatable logic, move from freeform spreadsheets to structured templates or cloud-native applications.
Implement AI guardrails
As we start to implement AI agents, introduce a “stop work authority” mechanism where any AI agent can halt processes if they detect anomalies beyond tolerance. This ensures human oversight remains responsive, not reactive.
Safeguard human judgement
Build explicit roles for human oversight into your AI architecture. Define clear boundaries where strategic, ethical, or high-risk decisions require human review. This ensures finance remains not just fast, but accountable — and keeps humans firmly at the helm, where it matters most.
Getting Future Ready isn’t about perfection — it’s about progress. The companies that start embedding structure, context, and oversight today will be the ones ready to scale with AI tomorrow. The transformation is already underway. The real question is: will finance lead it, or lag behind it?
Looking Further Ahead
CFOs don’t just manage today — they anticipate tomorrow. And just over the horizon is a new wave of change: Artificial General Intelligence.
Former OpenAI researcher Daniel Kokotajlo’s AI 2027 scenario outlines just how quickly we might see superintelligent AI emerge. Systems capable of autonomous coding and self-directed research could soon push AI beyond human intelligence—potentially automating their own progress and bringing superintelligence within reach.
When the next inflection point hits, finance won’t just be asked to keep up — it’ll be expected to lead.