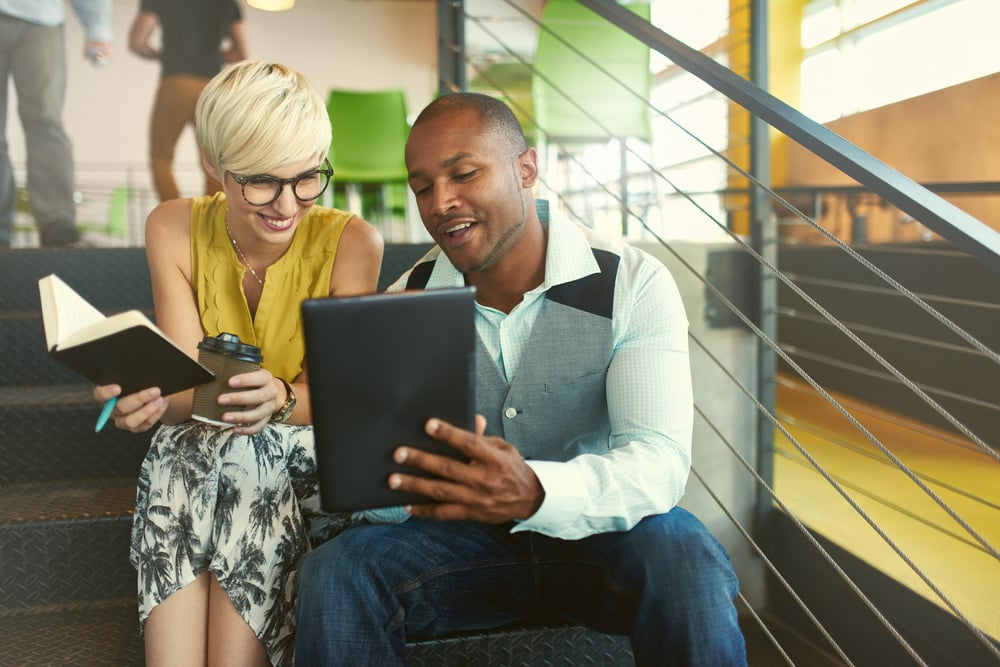
A Deeper Look at Agentic AI and the Future of Finance

What is Agentic AI?
Agentic AI isn’t just another chatbot or a smarter generative model with a few plugins. It represents a shift in how we structure workflows, moving from reactive tools to systems that plan intelligently, adapt to change, and deliver outcomes with minimal human input.
A helpful way to think about this is through the lens of cars. Basic cruise control keeps your speed steady, but you're still doing the thinking; steering, braking, reacting. Then came adaptive cruise control and emergency braking, which respond to the environment by adjusting speed or applying brakes when needed. These are like early forms of Autonomous AI Agents. Focused, goal-based, and single-purpose.
Now take that up a level. Self-driving cars integrate dozens of subsystems like navigation, braking, steering, lane assist and coordinate them in real time to handle the full driving experience end-to-end. These orchestrated systems resemble what we call orchestrator agents in AI: AI agents that sequence and manage multiple actions reliably and efficiently to achieve a larger goal, like running a full month-end close in finance.
Of course, the technology inside a self-driving car is far more complex than this analogy suggests. But it’s a useful way to grasp how orchestrated AI agents work together in practice.
But it doesn't stop there. Imagine those cars also communicating with each other, sharing road conditions, coordinating lane changes, and adjusting routes collectively to improve traffic flow and avoid collisions. This is how interacting agents operate: not just working inside one car, but collaborating across all cars to optimise outcomes for the entire network.
Types of AI Agents in Finance
We’ve already explored how agentic systems evolve from narrow, rule-based automation to intelligent orchestration and cross-system collaboration. Let’s bring that into a finance context.
1. Task Agents: Reliable Specialists
Task agents are your dependable, focused helpers. They perform straightforward, repeatable tasks—like checking for errors in reports, tagging journal entries, or routing documents to the right folder. They’re fast, cost-effective, and consistent, but only as capable as the specific instruction they’re given.
2. Autonomous Agents: Self-Guided Problem Solvers
Autonomous agents take it further. These are the self-steering systems that can handle multi-step processes on their own. For example, an autonomous agent might reconcile a ledger, spot irregularities, and draft a summary explanation—adjusting its actions based on the data it sees. It doesn’t just follow rules. It interprets the situation.
3. Orchestrator Agents: Digital Project Managers
Where autonomous agents manage individual tasks, orchestrator agents run the whole show. Like the self-driving car coordinating braking, navigation, and sensors, orchestrator agents manage multiple moving parts across a workflow. In finance, this could mean overseeing your full month-end close, from ingesting the data to preparing the finance report that goes to the board.
4. Interacting Agents: Collaborators Beyond Your Walls
Interacting agents go beyond the four walls of your business. They don’t just work across systems internally, they talk to other agents, systems, or organisations. Think of cars that coordinate lane changes with each other to ease congestion. In finance, this might eventually look like agents sharing documents with the auditors, or handling billing and payments with customers. These interactions create smoother collaboration and reduce human bottlenecks.
Together, these categories form a spectrum of capability—from narrow automation to adaptive, goal-oriented collaboration.
The Evolution of Single Agents to Ecosystems - Sooner Than You Think!
So far, most businesses have only experienced the earliest forms of agentic AI—task agents and simple autonomous agents embedded in familiar enterprise tools. Take Salesforce’s Agentforce, for example: service agents there can retrieve customer data, review billing history, and draft responses to inquiries. These aren’t chatbots—they’re purpose-built agents that quietly remove manual work from the workflow. When used well, they deliver real productivity gains with minimal disruption.
But we’re on the brink of something more powerful. We’re starting to see the foundations being laid for orchestrator-level agents, systems that can coordinate multiple agents, manage full workflows, and adapt in real time. And big tech players are clearing the path.
Google is leading this shift with its newly released Agent Builder and Agent Development Kit. These tools let developers build multi-agent systems and plug them into enterprise environments.
What makes this especially powerful is the infrastructure Google is building it on. Two open standards—Model Context Protocol (MCP) and Agent2Agent (A2A)—are designed to make interoperability simple. MCP introduced by Anthropic acts like USB-C for AI agents: it gives them a universal way to connect to your existing systems, from CRMs and ERPs to custom internal tools. Meanwhile, A2A introduced by Google, is like an API for agents—it lets them talk to each other regardless of their underlying frameworks or vendors.
This matters because it could enable you to assemble your own ecosystem—choosing the best agent for each task, plugging them into your existing systems, and letting them work together seamlessly.
Google has already launched an Agent Marketplace, with out-of-the-box agents from partners like Deloitte. The initial focus is on marketing and data use cases, but the implications go much wider. These agents won’t replace your core systems—they’ll enhance them, handling targeted workflows with more flexibility and less manual effort.
We’re still early—but the infrastructure is here, and the shift is underway.
Practical Steps for Finance Teams Today
To prepare your finance team without overinvesting, focus on low-risk foundational moves:
- Modularise workflows: Structure processes so individual steps can be automated by agents.
- Optimise your data: Ensure data cleanliness and clarity to maximise an agent’s ability to act effectively.
- Pilot simple task agents: Use tools like report accuracy checkers to build familiarity and confidence.
- Monitor your current software stack: Track how your existing platforms like Salesforce, NetSuite, or Workday are integrating agent-based capabilities.
- Stay alert to agent-driven SaaS alternatives: Generic AI agents are already replicating certain functions of legacy SaaS tools—like invoice processing or expense management. Although not yet fully enterprise-ready, these agents may begin to pressure traditional software providers on cost and flexibility. In essence, what you buy today might be built in-house tomorrow, faster, cheaper, and with greater control.
- Keep it practical: The best agent isn’t always the most advanced—it’s the one that reliably gets the job done. In many cases, that might still be a non-agent solution. Choose based on fit, not novelty.
AI Agents in Finance: Closer Than You Think
You won’t need to deploy a dozen agents tomorrow, but you may interact with them sooner than you expect. The best thing finance teams can do now is to stay informed, build flexible, agent-ready processes, and stay ready to act when the opportunity fits.
The shift is already underway and the teams that move steadily, not suddenly, will be best placed to benefit.
Next Steps
If you haven’t read our foundational article yet, start here: Finance and Agentic AI.
Curious what an autonomous month-end AI agent looks like in action? Check out: An AI Agent That Does Month-End Autonomously? Here's How I Built One to Prove It's Possible